Use cases
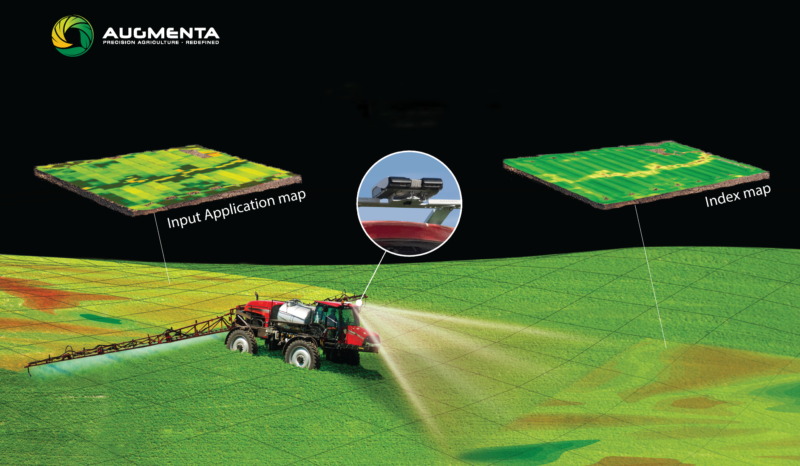
Smart Agriculture
The most promising solution for achieving higher yields in agricultural products with fewer chemicals is precision agriculture. The current reactive and low-precision technologies which perform operations using an experience- rather than a data-driven “blanket approach” do not address the issue. Augmenta (one of MLSysOps partners) offers an on-tractor fully automated, low-cost system for the monitoring and full lifecycle management (e.g., for different chemical inputs such as fertilizers and pesticides) of multiple different crops.
In MLSysOps, the system will be extended to synergistically operate in real-time with a scanning system-carrying, autonomous UAV (cooperation of multiple smart edge devices). Imaging from a UAV can offer a totally different perspective from the top which can be advantageous under specific external conditions (sunrise/sunset, glares on the field, high height crops, etc.). Furthermore, AI-driven control will be used to (i) manage the software pipeline and the mapping of software modules on the heterogeneous system according to the crops, operation, and external conditions, (ii) selectively deploy the drone, according to conditions and available battery capacity, (iii) partition the computational pipeline between the on-tractor device and the drone, (iv) control network connectivity between the on-tractor device and the drone, (v) manage the storage space on the device, the type of data stored according to free capacity (raw data or detected areas of interest) and the encoding, (vi) manage data staging towards the cloud, and (vii) manage ML training tasks.
Smart Cities
Experts at the World Health Organization report that 60% of people worldwide will live in urban areas by 2050. To make streets and sidewalks safer for pedestrians, cities can use real-time data from CCTV cameras and other sensors (noise, pollution, temperature, humidity, etc.) to better understand the liveliness of the city, to analyze how demand patterns change, to identify emergencies and provide faster response, and to produce complex analytics (for example correlating traffic congestion with pollution). Sensors can be either stationary or mobile (for example on public transport). Edge processing reduces both latency and OPEX costs (by avoiding the cost of the considerable amount of bandwidth needed to stream all videos to a centralized cloud location). The smart city use case provided by Ubiwhere will use 3 of the available smart lampposts in the city of Aveiro, featuring powerful NVIDIA Jetson edge nodes, to provide tracking solutions, crisis identification, and traffic congestion detection between the railway station and a specific road.
Manually managing such cloud-edge infrastructure is cumbersome, and requires a full view of the system status and goals and a high level of technical knowledge to understand and deal with the heterogeneous technologies. MLSysOps will both alleviate management burdens and improve its efficiency. The data communication paths and pre-processing of data at the deep-edge nodes will be optimized according to the status of the communication links of the sensor network and the available processing power and memory on these devices. Moreover, ML will optimize functionality to avoid wasting energy, storage, and processing capacity. ML is essential to optimize sensors and camera functionality based on historical data and, if necessary, change sensors and camera functionality if a new event is detected.
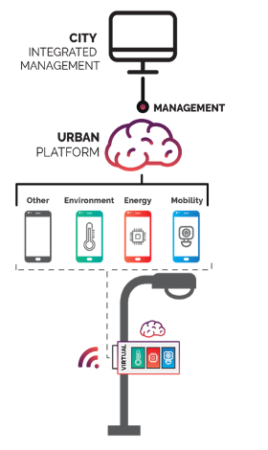